NVIDIA Nemotron: Agentic AI Advances
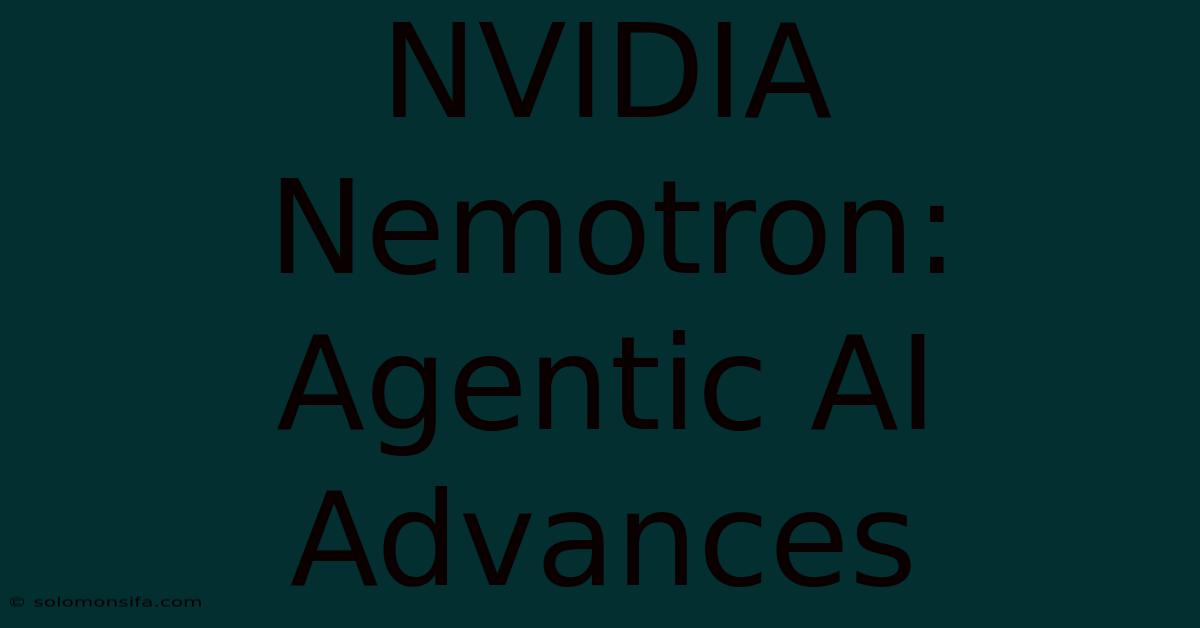
Discover more detailed and exciting information on our website. Click the link below to start your adventure: Visit Best Website nimila.me. Don't miss out!
Table of Contents
NVIDIA Nemotron: Unveiling the Next Generation of Agentic AI
Editor's Note: NVIDIA's Nemotron represents a significant leap forward in AI. This article explores its groundbreaking advancements and implications.
Why This Matters
The field of artificial intelligence is rapidly evolving, with a particular focus on creating more autonomous and intelligent systems. NVIDIA's Nemotron framework is a key player in this evolution, pushing the boundaries of agentic AI – AI systems that can act independently and pursue goals in complex environments. This review delves into the key features and implications of this transformative technology, encompassing its architecture, capabilities, and potential impact on various industries. We will explore its relationship to reinforcement learning, large language models, and the future of autonomous agents. Understanding Nemotron is crucial for anyone following advancements in AI and its potential applications.
Key Takeaways of Nemotron
Feature | Description |
---|---|
Agentic Capabilities | Enables AI to act autonomously and pursue goals. |
Reinforcement Learning Integration | Leverages RL for training agents to interact effectively with environments. |
Large Language Model Integration | Combines the power of LLMs for complex decision-making and reasoning. |
Scalability | Designed for deployment across various scales and resource constraints. |
Versatility | Adaptable to a wide range of applications and domains. |
NVIDIA Nemotron: A Deep Dive
Introduction: Nemotron represents a significant advancement in creating truly autonomous AI agents. Its architecture combines reinforcement learning (RL) and large language models (LLMs) to enable agents to learn, reason, and act effectively in complex, dynamic environments.
Key Aspects:
- Reinforcement Learning Integration: Nemotron leverages RL to allow agents to learn optimal strategies through trial and error. The agent receives rewards or penalties for its actions, guiding its learning process.
- Large Language Model Integration: The integration of LLMs provides the agent with advanced reasoning and decision-making capabilities. LLMs process information, interpret context, and formulate plans, enhancing the agent's overall intelligence.
- Scalability and Adaptability: Nemotron is designed for scalability, enabling its deployment in various environments and resource settings. Its adaptability allows it to be applied to diverse problems and domains.
Reinforcement Learning in Nemotron
Introduction: Reinforcement learning plays a crucial role in Nemotron, enabling agents to learn through interaction with their environment.
Facets:
- Reward System: The design of the reward system is critical; it must effectively guide the agent towards desired behaviors. A poorly designed reward system can lead to unintended or suboptimal outcomes.
- Exploration vs. Exploitation: Agents must balance exploring new actions and exploiting known effective actions. Finding the right balance is crucial for efficient learning.
- Training Environment: The complexity and realism of the training environment directly impact the agent's performance in real-world scenarios. A well-designed training environment allows the agent to generalize its skills effectively.
- Risks and Mitigation: Potential risks include unintended consequences due to flawed reward functions or insufficient training. Mitigation strategies include rigorous testing, careful reward system design, and robust error handling.
- Impact: The impact of reinforcement learning in Nemotron is the creation of agents capable of adapting and learning in dynamic situations, mimicking human adaptability and skill development.
Large Language Models and Agentic AI
Introduction: The integration of LLMs significantly enhances the capabilities of Nemotron agents. LLMs provide the agents with a higher level of cognitive function, enabling more complex decision-making and problem-solving.
Further Analysis: LLMs allow the agent to interpret natural language instructions, reason about complex situations, and plan sequences of actions effectively. For example, an LLM integrated into a robotic agent might interpret a command like "Clean the kitchen" and plan a series of actions, such as gathering cleaning supplies, wiping counters, and washing dishes, all based on its understanding of the environment and task.
Closing: The synergy between RL and LLMs within Nemotron creates a powerful combination, resulting in agents that can not only learn through experience but also reason strategically, ultimately leading to more advanced and versatile AI systems. Challenges remain in managing the complexity and computational demands of such a system, but the potential benefits are substantial.
Nemotron Capabilities: A Comparative View
Feature | Nemotron | Traditional RL Agents |
---|---|---|
Reasoning | Advanced via LLM integration | Limited |
Planning | Strategic planning capabilities | Reactive or simple planning |
Natural Language | Understands and responds to instructions | Limited or none |
Adaptability | High, adapts to diverse environments | Can be limited |
Complexity | Handles complex tasks and environments | Often simpler tasks |
FAQ
Introduction: This section addresses common questions about NVIDIA Nemotron.
Questions:
-
Q: What is the main difference between Nemotron and other agentic AI systems? A: Nemotron's unique strength lies in the synergistic combination of reinforcement learning and large language models, allowing for far more complex reasoning and planning capabilities.
-
Q: What are the potential applications of Nemotron? A: Potential applications span robotics, autonomous driving, virtual assistants, and more, enabling sophisticated autonomous agents across multiple sectors.
-
Q: What are the limitations of Nemotron? A: Significant computational resources are required, and the training process can be computationally expensive and time-consuming.
-
Q: How does Nemotron address ethical concerns in AI? A: While the framework itself doesn't directly address ethics, its design necessitates careful consideration of reward functions and training environments to avoid unintended biases and harmful outcomes.
-
Q: Is Nemotron open-source? A: Currently, information about open-source availability is not publicly accessible.
-
Q: What are the future development prospects for Nemotron? A: Future development likely involves enhancing the efficiency of training processes, improving robustness, and expanding its applicability to more complex real-world scenarios.
Summary: The FAQ section highlights the key features, limitations, and future prospects of Nemotron, clarifying common questions and addressing potential concerns.
Tips for Utilizing Nemotron
Introduction: This section provides tips for effectively leveraging the capabilities of Nemotron.
Tips:
-
Carefully design your reward function: A well-designed reward function is crucial for guiding the agent effectively. Avoid unintended biases or rewarding undesired behaviors.
-
Choose the right training environment: Select a training environment that accurately reflects the real-world scenario where the agent will operate.
-
Monitor agent performance closely: Continuously monitor the agent's performance and adjust the training process as needed.
-
Utilize appropriate safety mechanisms: Implement safety mechanisms to prevent harmful actions or unintended consequences.
-
Embrace iterative development: Iterative development and testing are crucial for refining the agent's behavior and capabilities.
-
Leverage advanced monitoring tools: Utilize advanced monitoring tools to gain a comprehensive understanding of the agent's learning process.
Summary: These tips highlight the importance of careful design, monitoring, and iterative development in utilizing Nemotron effectively.
Summary of NVIDIA Nemotron
Summary: This article explored NVIDIA's Nemotron, a groundbreaking framework for developing agentic AI systems. The integration of reinforcement learning and large language models represents a major advancement, enabling the creation of autonomous agents capable of complex reasoning, planning, and adaptation. While challenges remain, the potential applications and implications of Nemotron are significant, shaping the future of AI across various industries.
Closing Message: Nemotron showcases the rapid progress in agentic AI. As the technology continues to evolve, it's crucial to consider the ethical implications and ensure responsible development and deployment, maximizing its benefits while mitigating potential risks. The future of AI is being shaped by such innovative advancements, promising transformative possibilities for the years to come.
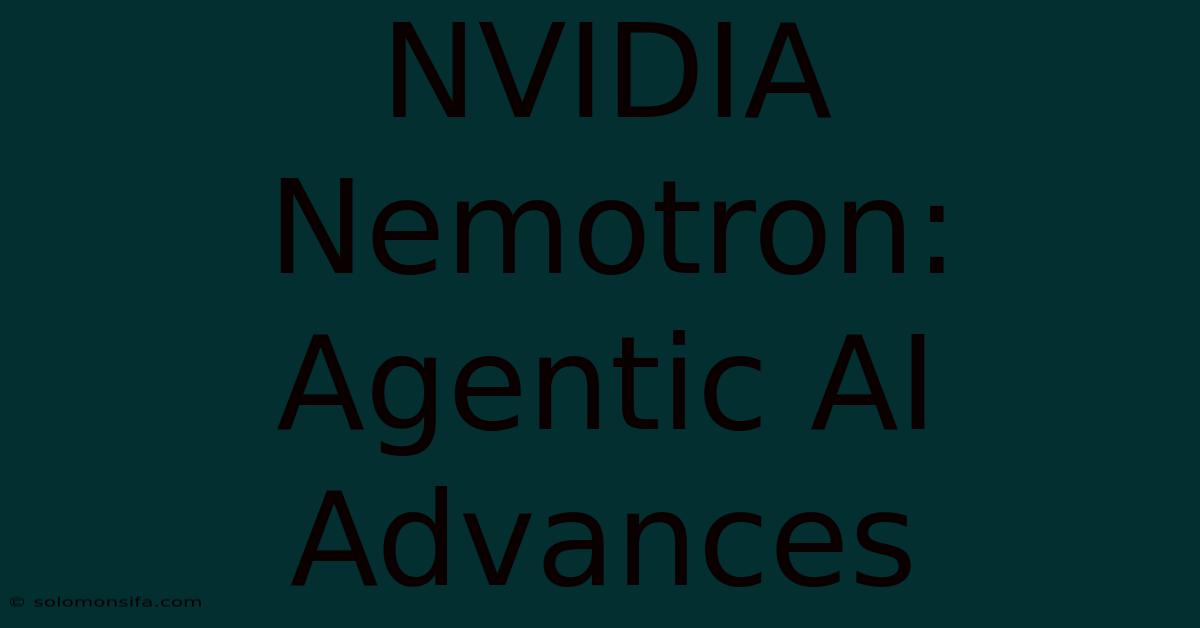
Thank you for visiting our website wich cover about NVIDIA Nemotron: Agentic AI Advances. We hope the information provided has been useful to you. Feel free to contact us if you have any questions or need further assistance. See you next time and dont miss to bookmark.
Featured Posts
-
Spanish Press On Alexander Arnold
Jan 07, 2025
-
Nvidia Stock Plunges After Record High
Jan 07, 2025
-
Omniverse Nvidias Industrial Design
Jan 07, 2025
-
Worst Golden Globes Winner Ever
Jan 07, 2025
-
Nvidia Unveils Industrial Omniverse
Jan 07, 2025