NVIDIA Cosmos World Model Platform Launch
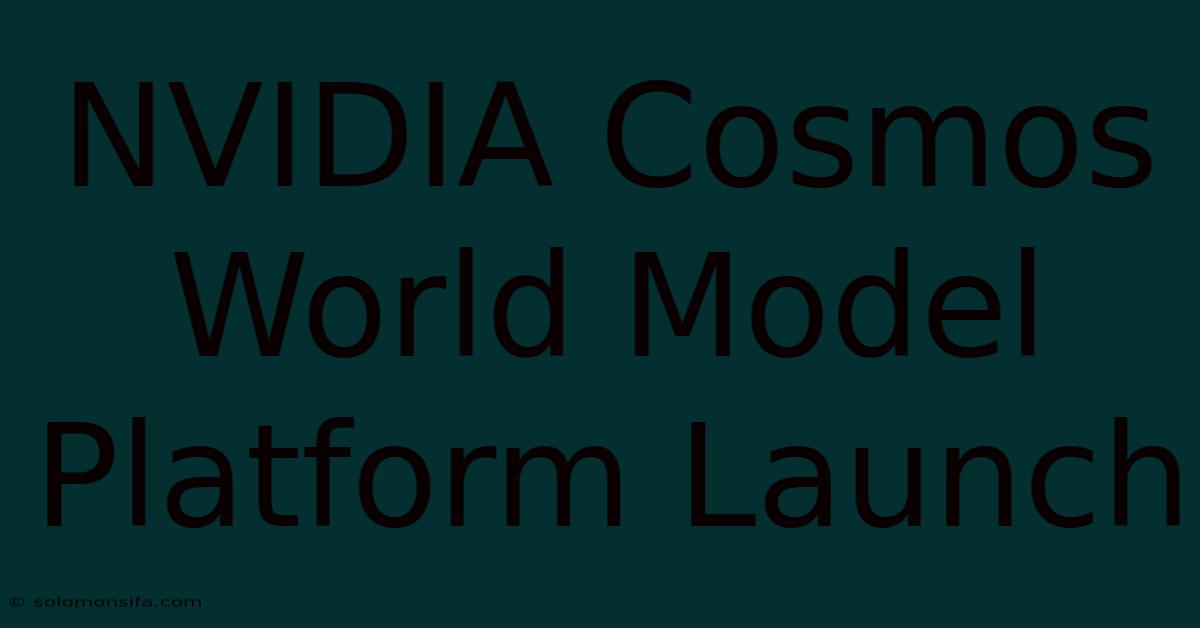
Discover more detailed and exciting information on our website. Click the link below to start your adventure: Visit Best Website nimila.me. Don't miss out!
Table of Contents
NVIDIA Cosmos: Unveiling a New Era in World Models
Editor's Note: NVIDIA's launch of the Cosmos world model platform marks a significant advancement in AI. This article explores its key features and implications.
Why It Matters
The release of NVIDIA's Cosmos platform is a pivotal moment in AI development. This innovative system promises to revolutionize how AI interacts with and understands the world, paving the way for more sophisticated and adaptable AI agents. This review delves into the core functionalities of Cosmos, highlighting its potential impact on various industries and addressing potential challenges. We will explore topics like generative AI, large language models, simulation, and the broader implications for AI safety and ethical considerations. Semantic keywords like "AI simulation," "generative AI models," "synthetic data," and "world modeling" are central to understanding the significance of this launch.
Key Takeaways of NVIDIA Cosmos
Feature | Description | Impact |
---|---|---|
Generative AI | Creates realistic simulations and synthetic data. | Enables training of robust AI models in diverse scenarios. |
Large Language Models (LLMs) Integration | Seamlessly integrates with LLMs for enhanced reasoning and contextual understanding. | Allows for more nuanced and intelligent AI agent interactions. |
Physics Simulation | Accurately models physical laws and interactions within the simulated world. | Leads to more realistic and predictable AI behavior in real-world applications. |
Scalability | Designed for efficient scaling to handle complex simulations and large datasets. | Enables training of more sophisticated and capable AI models. |
NVIDIA Cosmos World Model Platform
Introduction
NVIDIA Cosmos represents a significant leap forward in AI's ability to understand and interact with complex environments. This platform utilizes a combination of advanced technologies to create highly realistic and dynamic simulated worlds, allowing for the training and testing of AI agents in a safe and controlled environment.
Key Aspects
- Generative AI Capabilities: Cosmos leverages powerful generative AI models to create realistic and diverse simulated environments, significantly enhancing the training data available for AI agents.
- Physics Engine Integration: A robust physics engine ensures that the simulated world adheres to the laws of physics, leading to more accurate and predictable AI behavior.
- Large Language Model (LLM) Integration: Cosmos seamlessly integrates with LLMs, enabling AI agents to understand and respond to complex textual inputs and generate human-like text.
- Scalability and Performance: The platform is designed for scalability, allowing researchers and developers to train and test AI models on a vast scale.
Discussion
The combination of generative AI, a sophisticated physics engine, and LLM integration within Cosmos creates a unique environment for training AI agents. This allows for the development of AI systems capable of navigating complex real-world scenarios with greater adaptability and robustness. The scalability aspect ensures that these models can be trained and tested on a large scale, unlocking the potential for significant breakthroughs in various fields, including robotics, autonomous driving, and virtual reality.
The Role of Generative AI in NVIDIA Cosmos
Introduction
Generative AI plays a crucial role in creating the rich and diverse simulated worlds within the Cosmos platform. It's the engine that generates the data and visual elements that make the simulations realistic and effective for training AI agents.
Facets
- Data Generation: Generative models produce vast amounts of training data, overcoming limitations of real-world data scarcity.
- Variety and Complexity: Generative models allow for creation of scenarios with varying levels of complexity and unpredictability, fostering adaptable AI.
- Risks: Potential for bias in generated data, leading to biased AI agents. Requires careful monitoring and mitigation strategies.
- Mitigation: Implementing robust data validation and bias detection mechanisms is crucial. Diverse training datasets are also essential.
- Impacts: Improves the quality and robustness of trained AI agents, enabling safer and more effective deployment in real-world applications.
Summary
Generative AI ensures Cosmos provides a diverse and realistic training environment, directly impacting the performance and reliability of the AI agents developed using the platform. Addressing the risks associated with biased data generation is paramount for responsible AI development.
The Importance of Physics Simulation in NVIDIA Cosmos
Introduction
Accurate physics simulation is critical for creating believable and reliable virtual environments within the Cosmos platform. This ensures that AI agents trained within these environments translate their learned behaviors effectively to the real world.
Further Analysis
Consider the scenario of training a self-driving car. Without accurate physics simulation, the AI might learn behaviors that are effective in the simulated world but unsafe in the real world due to inaccuracies in how physics is modeled.
Closing
Precise physics modeling is non-negotiable for training robust and dependable AI agents in the context of Cosmos. The accuracy of the simulation directly translates into the reliability and safety of the AI systems developed using this platform. This underscores the importance of rigorous testing and validation of the physics engine within Cosmos.
Key Insights on NVIDIA Cosmos (Information Table)
Aspect | Description | Benefit | Challenge |
---|---|---|---|
Generative AI | Creates varied and realistic simulated environments. | Enhances training data and improves AI agent adaptability. | Potential for bias in generated data. |
Physics Simulation | Accurate modeling of physical laws. | Leads to more reliable and safe AI agent behavior in real-world scenarios. | Computational cost and complexity. |
LLM Integration | Seamless integration with Large Language Models. | Enables more complex and nuanced AI agent interactions. | Ensuring coherent interaction between LLM and simulated environment. |
Scalability | Ability to handle large-scale simulations and datasets. | Enables training of more sophisticated AI models. | Managing computational resources and maintaining performance. |
Ethical Considerations | Addressing potential biases and ensuring responsible AI development. | Promotes trustworthy and beneficial AI applications. | Continuous monitoring and refinement of ethical guidelines. |
FAQ
Introduction
This section addresses frequently asked questions regarding the NVIDIA Cosmos platform.
Questions
- Q: What types of AI agents can be trained using Cosmos? A: A wide range, including robotics agents, autonomous vehicle agents, and virtual character agents.
- Q: How does Cosmos address AI safety concerns? A: Through the use of controlled simulated environments and rigorous testing procedures.
- Q: What are the computational requirements for using Cosmos? A: Significant computational resources are required, ideally high-performance computing clusters.
- Q: Is Cosmos accessible to individual researchers? A: Accessibility may depend on resource availability and collaborations with NVIDIA.
- Q: What are the potential applications of Cosmos beyond research? A: Numerous industries can benefit, including manufacturing, healthcare, and entertainment.
- Q: How does Cosmos handle data privacy and security? A: NVIDIA employs robust security measures to protect data within the platform.
Summary
The FAQs highlight the versatility, safety features, and resource requirements associated with NVIDIA Cosmos. Addressing ethical concerns and data security are integral to the platform’s design.
Tips for Utilizing NVIDIA Cosmos
Introduction
This section provides guidance for effectively leveraging the Cosmos platform.
Tips
- Start with simple simulations: Begin with less complex scenarios to become familiar with the platform.
- Utilize pre-built assets: Take advantage of any available pre-built assets to accelerate development.
- Monitor performance metrics: Closely observe key metrics to identify areas for optimization.
- Iterate and refine: Continuous iteration and refinement are key to success with any AI project.
- Collaborate with the community: Engage with the NVIDIA developer community to learn from others' experiences.
- Focus on data quality: High-quality data is essential for training effective AI agents.
- Employ robust testing procedures: Thorough testing is crucial for ensuring the reliability and safety of your AI agents.
Summary
These tips emphasize a gradual approach, leveraging available resources, and prioritizing data quality and testing to maximize the benefits of the NVIDIA Cosmos platform.
Resumen de NVIDIA Cosmos
Resumen: Este artículo explora la plataforma de modelos mundiales NVIDIA Cosmos, destacando sus capacidades de IA generativa, simulación física y la integración de modelos de lenguaje extenso. Se analizan los aspectos clave, incluyendo la generación de datos, la mitigación de riesgos y las implicaciones éticas. Se presentan consejos para el uso eficaz de la plataforma y se responden preguntas frecuentes.
Mensaje final: El lanzamiento de NVIDIA Cosmos representa un avance significativo en el campo de la IA. Su capacidad para crear simulaciones realistas y entrenar agentes de IA robustos abre nuevas posibilidades en diversos sectores. La adopción responsable y la consideración de las implicaciones éticas son cruciales para aprovechar al máximo su potencial.
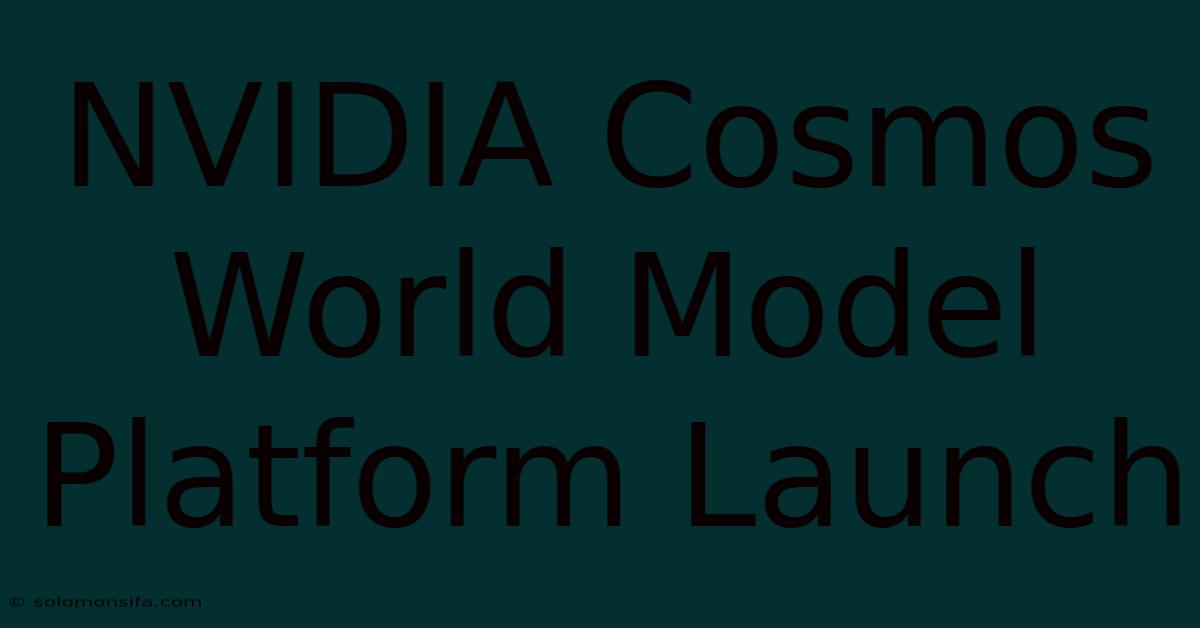
Thank you for visiting our website wich cover about NVIDIA Cosmos World Model Platform Launch. We hope the information provided has been useful to you. Feel free to contact us if you have any questions or need further assistance. See you next time and dont miss to bookmark.
Featured Posts
-
Shigatse Earthquake Latest Updates
Jan 07, 2025
-
Maintain Microsoft 365 Winter Grad Action
Jan 07, 2025
-
Carabao Cup Semi Final Expert Q And A
Jan 07, 2025
-
All Stars Love Island 2025 Confirmed Lineup
Jan 07, 2025
-
Arnold Transfer Real Madrids Challenge
Jan 07, 2025