Nemotron Model Families: NVIDIA's AI Leap
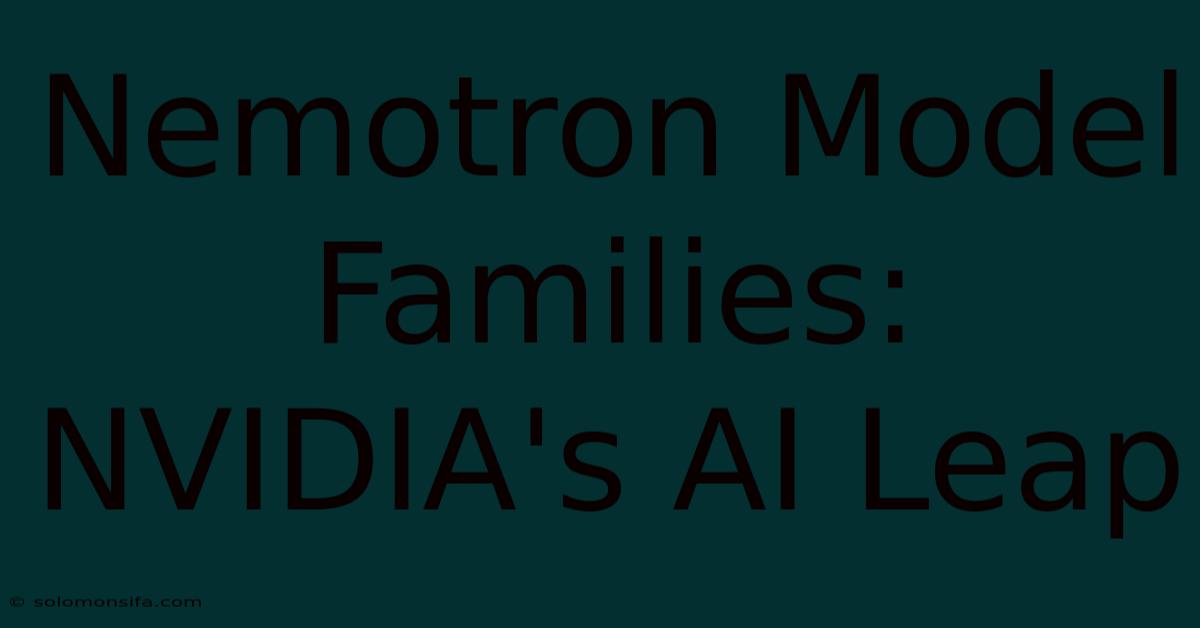
Discover more detailed and exciting information on our website. Click the link below to start your adventure: Visit Best Website nimila.me. Don't miss out!
Table of Contents
Nemotron Model Families: NVIDIA's AI Leap
Editor's Note: NVIDIA's Nemotron model families have been released, marking a significant advancement in AI.
Why It Matters
Understanding NVIDIA's Nemotron model families is crucial for anyone involved in AI development, deployment, and application. This review explores the key features, benefits, and implications of these powerful new models, providing insights into their potential to revolutionize various industries. We will delve into their architecture, capabilities, and potential impact on large language models (LLMs), speech AI, and more. Keywords such as Nemotron, NVIDIA, AI models, large language models, speech AI, generative AI, and deep learning will be explored throughout.
Key Takeaways of Nemotron
Feature | Description |
---|---|
Scalability | Designed for efficient training and deployment across various hardware. |
Performance | Delivers state-of-the-art results in various AI tasks. |
Versatility | Supports a wide range of applications, including LLMs and speech AI. |
Customization | Allows for fine-tuning and adaptation to specific needs and datasets. |
Ecosystem | Integrates seamlessly with NVIDIA's broader AI platform and tools. |
Nemotron Model Families
Introduction
NVIDIA's Nemotron model families represent a significant leap forward in AI capabilities. These models are designed to be highly scalable, performant, and versatile, enabling developers to build and deploy cutting-edge AI applications across diverse domains.
Key Aspects
Nemotron models are characterized by several key aspects that contribute to their superior performance and adaptability:
- Modular Architecture: This allows for easier customization and scalability.
- Optimized for NVIDIA Hardware: Nemotron models are designed to leverage the full potential of NVIDIA's GPUs and other hardware, resulting in significant performance gains.
- Pre-trained Models: Availability of pre-trained models accelerates development and reduces training time.
- Support for Multiple Modalities: Nemotron supports various data types, including text, audio, and images.
Discussion
The modular architecture of Nemotron allows developers to select and combine different components to create custom models tailored to specific tasks. This flexibility is crucial for addressing the unique requirements of various AI applications. The optimization for NVIDIA hardware ensures efficient training and deployment, minimizing computational costs and maximizing performance. The availability of pre-trained models significantly reduces development time and resources, allowing developers to focus on fine-tuning and application-specific adjustments. The support for multiple modalities broadens the scope of applications, enabling the creation of powerful multimodal AI systems.
The Connection Between Efficient Training and Nemotron
Introduction
Efficient training is paramount in the development and deployment of large-scale AI models. Nemotron's architecture directly addresses this challenge.
Facets
- Role of Parallel Processing: Nemotron leverages parallel processing capabilities of NVIDIA GPUs to drastically reduce training time.
- Examples of Efficiency: Training time reductions compared to previous generations of models serve as strong examples.
- Risks of Inefficient Training: High computational costs and prolonged development cycles are significant risks.
- Mitigation Strategies: Nemotron's design incorporates several mitigation strategies to minimize these risks.
- Impact on Deployment: Efficient training leads to faster deployment and reduced operational costs.
Summary
The efficient training enabled by Nemotron’s architecture is a key factor in its success. It facilitates the creation of larger and more complex AI models without sacrificing development time or resources.
The Importance of Customization in Nemotron
Introduction
Customization allows users to adapt Nemotron models to their unique needs and datasets. This is a crucial aspect of building effective and targeted AI solutions.
Further Analysis
The ability to fine-tune pre-trained Nemotron models for specific applications offers significant advantages. This reduces the need for extensive training from scratch, saving time and resources. Examples include customizing a Nemotron model for medical diagnosis using a specific hospital's dataset or adapting a model for fraud detection using a particular financial institution’s transaction data.
Closing
Customization is a critical feature of Nemotron that ensures its versatility and adaptability across a wide range of applications. It empowers developers to leverage the power of pre-trained models while tailoring them to meet specific business and research objectives, bridging the gap between general-purpose AI and specialized AI solutions.
Nemotron Model Families: Key Insights
Model Family | Key Features | Target Applications |
---|---|---|
Nemotron-LLM | Large language model capabilities | Chatbots, text summarization, translation |
Nemotron-Speech | Advanced speech recognition and synthesis | Voice assistants, transcription, speech therapy |
Nemotron-Vision | Image and video processing capabilities | Object detection, image classification, video analysis |
FAQ
Introduction
This section addresses frequently asked questions regarding NVIDIA's Nemotron model families.
Questions
-
Q: What hardware is required to run Nemotron models? A: Nemotron models are optimized for NVIDIA GPUs, but can potentially be adapted to other hardware with appropriate modifications.
-
Q: How do Nemotron models compare to other AI models? A: Nemotron models aim to offer superior scalability, performance, and customization options compared to many existing models. Benchmark comparisons will need to be made on a case-by-case basis.
-
Q: Are the Nemotron models open source? A: Information on the licensing and availability of the models needs to be obtained from official NVIDIA sources.
-
Q: What level of expertise is needed to use Nemotron models? A: Experience with deep learning and AI frameworks is beneficial, but NVIDIA likely offers resources and documentation to support different user levels.
-
Q: What are the potential ethical considerations of using Nemotron models? A: As with all powerful AI models, ethical considerations regarding bias, fairness, and responsible use must be carefully considered.
-
Q: What is the future roadmap for Nemotron model families? A: Further details on the future roadmap and updates should be sought from official NVIDIA announcements.
Summary
The FAQ section highlights the key aspects of Nemotron's accessibility, performance, and ethical considerations.
Tips for Utilizing Nemotron
Introduction
This section provides practical tips for effectively utilizing Nemotron model families.
Tips
- Start with pre-trained models: Leverage pre-trained models to accelerate development.
- Understand the modular architecture: Utilize the modularity for customization.
- Optimize for your hardware: Ensure your hardware is compatible and configured for optimal performance.
- Monitor performance metrics: Closely track performance metrics to fine-tune and optimize models.
- Experiment with different configurations: Test different model configurations to find the best fit for your application.
- Consider ethical implications: Always consider potential ethical implications.
- Stay updated: Keep abreast of the latest updates and improvements from NVIDIA.
Summary
These tips will help users get the most out of Nemotron model families.
Summary by Nemotron Model Families
This article explored NVIDIA's Nemotron model families, highlighting their key features, benefits, and applications. The modular architecture, optimized performance, and customization options make them a powerful tool for AI developers. Efficient training, adaptability, and consideration of ethical implications were key discussion points.
Mensaje de cierre (Closing Message)
NVIDIA's Nemotron model families represent a significant step towards more accessible and powerful AI. Their impact will be felt across various industries as developers leverage their capabilities to create innovative and impactful applications. The future of AI is bright, and Nemotron is paving the way.
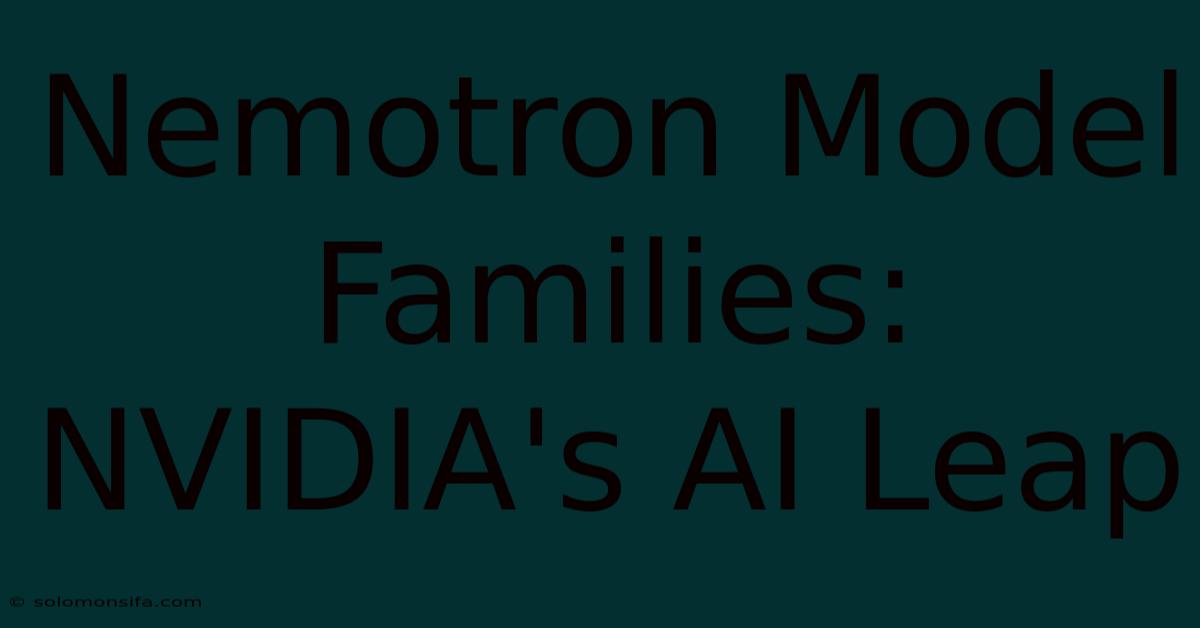
Thank you for visiting our website wich cover about Nemotron Model Families: NVIDIA's AI Leap. We hope the information provided has been useful to you. Feel free to contact us if you have any questions or need further assistance. See you next time and dont miss to bookmark.
Featured Posts
-
Wolves Team News Lima Semedo Injury Report
Jan 07, 2025
-
Deadly Tibet Earthquake Death Toll Rises
Jan 07, 2025
-
Wolves Forest Lima Debut Semedo Injury News
Jan 07, 2025
-
Microsoft 365 Access Winter Graduates
Jan 07, 2025
-
Real Madrid Starting Xi Deportiva
Jan 07, 2025